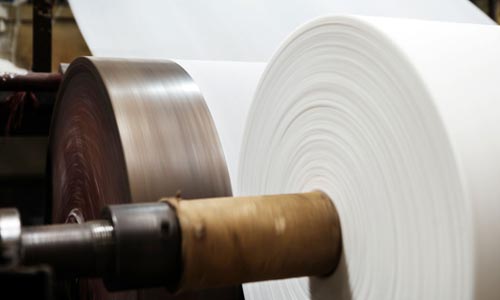
How does the Predictive Wet End service help prevent runnability and quality issues? What is required from the paper or board mill to get started with the service? Find out the answers to these and other common questions about our new digital service for paper and board below. And in case you missed out on our experts’ webinar discussion on the topic, you can register for the on-demand session here.
What is Predictive Wet End?
Kemira KemConnect™ Predictive Wet End is a new digital service for paper and board. The cloud-based service uses predictive analytics and machine learning to discover wet end chemistry related phenomena that can impact the paper or board machine’s runnability, performance or end-product quality.
For a long time, Kemira has developed real-time measuring and monitoring solutions to better understand the chemistry applications and to control and optimize the chemical programs at paper and board machines. Predictive Wet End is a step further, making it possible to predict and prevent instabilities and disturbances in the production process even hours before they happen.
What kind of production issues can the service predict?
Wet end chemistry impacts the paper and board production process in multiple complex ways. Stable wet end performance helps ensure overall machine efficiency and end-product quality. But without deep understanding of chemistry and visibility to the end-to-end process conditions, wet end stability is difficult to achieve.
The Predictive Wet End service can reveal previously unseen cause-and-effect relationships between different variables in the papermaking process and pinpoint process conditions and phenomena that result in runnability or quality problems. Based on data, it can predict the risk of e.g. end-product defects, deposits or web brakes and thus help prevent off-spec production or production downtime at the machine.
How are the predictions created?
The Predictive Wet End service efficiently combines the available process and performance data from the paper or board machine and e.g. from the on-line solutions for monitoring chemistry applications. Utilizing modern cloud computing and machine learning technology, the service can identify situations where the risk for production disturbances increases and alert the operators to take preventative action.
The predictive model is built on Kemira’s extensive experience on paper machine chemistry and applications and trained with each customer’s historical process data. Data can be collected from a few hundred up to thousands of data points, depending on the production process and the problem to solve.
How does Predictive Wet End service help prevent breaks or off-spec production?
The service can predict process conditions and performance up to 5 hours ahead and detect when the risk for production disturbances increases. The algorithm highlights which process areas and which parameters contribute to the increased risk and gives actionable recommendations on how to stabilize the wet end chemistry and the entire process. This way, the operators can make chemical and mechanical adjustments in the process even before the production is impacted. The insights, alerts and recommendations are made available via an easy-to-use user interface, a sort of a window to the future.
What kind of data is needed for the predictions?
The obvious starting point is that the data used needs to be reliable and easily available. The service can use data from multiple sources, such as the process and performance data from the machine, measurements from e.g. Kemira’s own on-line monitoring solutions, laboratory measurement data etc. The exact needed data tags are determined by the production issue to solve, but for example, real-time measurements on the chemical health of the process, such as pH, conductivity, and cationic charge, are important and help improve the reliability of the predictions.
The first step in a new project is always a process chemistry and data health check, where the usability of existing and available data is evaluated before moving forward with the implementation.
How long does it take before the model learns to predict the wet end performance at a new machine?
As the predictive model is based on a knowledge base that is built on years of expertise and experiences from multiple customer cases, it reliably identifies the most common chemistry related disturbances at paper and board machines. Every machine and every production environment is still different. The model is trained with each customer’s historical data to adapt to the specific circumstances. Depending on the case, it typically takes a couple of months.
What are the key benefits of the Predictive Wet End service?
The reliable predictions hours into the future enable fact-based and timely decisions in the mill operations. This improves the process stability and reduces and prevents e.g. runnability issues, end-product defects, and web breaks before they happen. The reduction of production downtime and rejected reels help improve the overall equipment efficiency (OEE) and bring about considerable annual cost savings.
More stable wet end can also mean improved performance of e.g. retention, strength, and sizing chemicals, leading to optimized chemistry and raw material usage and reduced cost.
What if the production process changes after the implementation of the service?
The service utilizes machine learning technology which means that the predictive model can adapt to new situations. Typically, the model is re-trained on a regular basis to ensure the reliability of the predictions, and it should be done whenever there is a major change in the production process to take into account possible new parameters and process variables.
What is required from the paper or board mill to get started?
It all starts with an identified production challenge to solve. In the first phase of the project, we perform a data and chemistry health check to validate the usability of the model, evaluate the data available, and define targets and KPIs. In many cases, already the chemistry health check exposes new root causes for production instabilities, providing valuable insight that helps improve the stability of the wet end chemistry.